Do Recommender Systems Mobilize People for Collective Actions? Is it Dependent on Recommenders’ Design or People’s Usage?
- Jin Wan
- Jul 30, 2024
- 3 min read
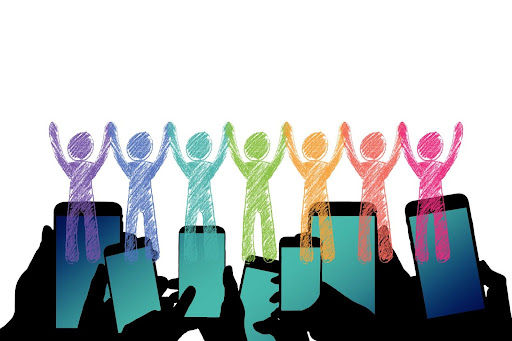
Tools and platforms that collective actions such as protests and parades rely on to mobilize, organize, and communicate with people have evolved tremendously due to technological advancement. One important change is the wide application of social media, which heavily incorporates algorithms to rank, filter, and disseminate content. This set of algorithms is called recommender systems (“RS” for short).
Digital activism researchers have noticed the role of RS in influencing collective actions, but most of them concentrate on the perspective of activists. Activists use RS content filtering and aggregation functions to facilitate collective actions on social media while trying to mitigate the constraints placed by algorithms such as information overload and content exclusion (Etter & Albu, 2021). Moreover, they consciously tailor their content strategies to RS on different platforms (Dumitrica & Felt, 2020).
However, it is yet to be known how activists using RS for collective actions might influence other people to act as a group, such as their feelings of connectedness, knowledge about the compaign, interest in the topic, etc.. Better understanding in this regard can help activists make more informed choices of digital tools and develop mobilizing strategies in collective actions.
To this end, my collaborators and I plan to look into the effects of people’s RS usage on factors that might influence people’s engagement in collective actions. We want to explore whether, and if any, how different RS mobilize people for collective actions. Platforms included in our study are mainly social media, such as Facebook, Instagram, X, etc., because RS are heavily incorporated in these platforms’ infrastructures.
We initially anticipated that the variation of RS effects might arise from differences in RS design across platforms. For example, some RS, such as those on Facebook, prioritize interactions users make with other accounts. It means that these RSFacebook prioritize the uses of likes, comments, sharing etc. for ranking and filtering content. While other RS, such as TikTok, give more weight to users’ own activities like watch history over their networks in creating recommendations (the “for me” page).
What we find tricky but also fascinating when we delve deeper into this idea is that the interaction between users and RS might complicate the categorization we proposed above. While RS on a platform are designed to be equipped with features enabling and encouraging users' connections, people might use this platform in a very solitary way, for example, never following anyone on Instagram. Put it another way, RS effects on mobilizing people might not only depend on their design but also potentially on how people use them.
How to take this interaction between users and RS into account when differentiating recommenders? Which one is more determinant in terms of RS effects on mobilization? If you have any thoughts (or any related topics) in this regard, feel free to shoot me a message and I am happy to discuss.
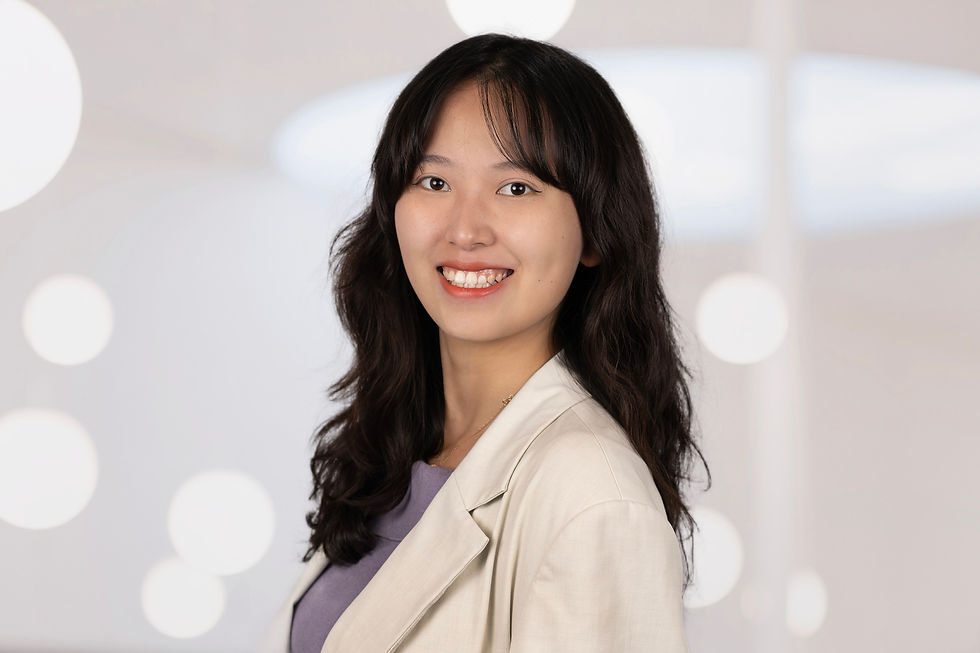
Jin Wan is a PhD candidate at the Amsterdam School of Communication Research (ASCoR). Her PhD project is part of the larger project Public Values in the Algorithmic Society (AlgoSoc). She is interested in usage of recommender systems in daily life and its influence on people’s information consumption and political behaviour.
Reference:
Dumitrica, D., & Felt, M. (2020). Mediated grassroots collective action: negotiating
barriers of digital activism. Information, Communication & Society, 23(13),
Etter, M., & Albu, O. B. (2021). Activists in the dark: Social media algorithms and
collective action in two social movement organizations. Organization (London,
England), 28(1), 68–91. https://doi.org/10.1177/1350508420961532
This post really made me rethink how I approach this topic. I never realized this detailed information could have such an impact. Thanks for shedding light on this! Being your fan, I want to grab your sight on my work on taurus and aquarius love compatibility. I request you to please have a look at our website and share your feedback with us.
Wow! Your blog was a fantastic read—so much valuable information. We’re currently working on content related to “zodiac sign by name”, and I’d love to know your perspective on it. Looking forward to more amazing content from you!
Your blog was truly insightful! I loved how you broke down the details so clearly. We're currently diving into the theme of 'zodiac sign birthstone' and would love to hear your perspective on it. Looking forward to your next piece!
Your blog post was incredibly informative! I was amazed by the level of detail and knowledge you shared. We are exploring the field of "february gemstone" and would truly value your suggestions. Please consider visiting our website for a glimpse of our work. Looking forward to your next blog and your valuable feedback!
Your blog post really captured my interest! I never realized how vast and insightful this topic could be. We are currently working on "aquarius and leo compatibility", and it would be wonderful to get your suggestions and insights. Please take a moment to visit our website for a quick overview of our work. Looking forward to your feedback and your next blog!