“Warm smile required”: What exactly are the machines learning?
- Noon Abdulqadir
- Mar 3, 2022
- 2 min read
In this blogpost, I will provide a brief overview of the main findings of the first paper of my PhD research.
When I’m asked about my research, I usually go with the most accessible description: “I study bias in AI recruitment tools”. What I skip is the part I think might be the most disconcerting: “… and this bias comes from us”.

Before we jump into the main findings of my study, you must know three things:
Employers target you on professional platforms such as LinkedIn. They do so implicitly, by writing job advertisements in a way that appeals to certain social groups. This manner of writing, called framing, may rely on stereotypes, and we know that machine learning-based algorithms can pick up on textual features.
Broadly, social groups are viewed as either warm or competent. Women are expected to be warm and men to be competent.
We observe a gender-equality paradox. This means that while we see many countries becoming more egalitarian (so: fostering more equality and equal treatment across gender, religion, economic status, political beliefs, etc.), gender gaps and stigma in jobs have loosened but seem to stagnate now. And this paradox has become a staple for public argumentations. Infamously, Jordan Peterson – Canadian clinical psychologist, YouTube personality, and author – repeats this point again, and again, and again.
Under the premise that job advertisements from sectors where one social group dominates are likely to target members of that social group and are framed in a way that aligns with the stereotypes of that group, we expect job ads from female-dominated sectors to contain more warmth frames and those from male-dominated sectors to contain more competence frames. We detected these frame differences using machine learning algorithms. The underlying idea here was: if our models can find these differences, recruitment tools definitely will.
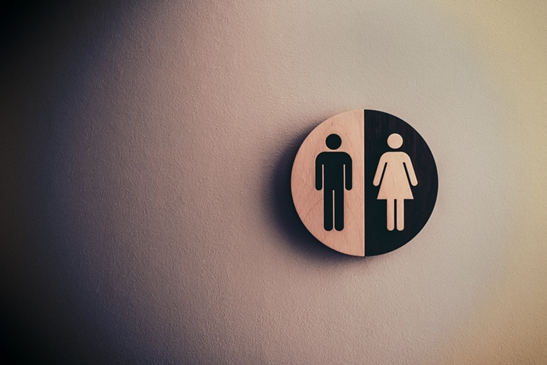
So, how did it go? We detected frame differences with an accuracy of 78%, which means we’re pretty certain recruitment tools can as well. Researchers have matched positions to candidates using job ad content, and with companies like Amazon and LinkedIn exploiting textual content to inform hiring decisions, we expect job ad framing to influence candidate pools tremendously in the near future.
What’s more, our findings show the egalitarian gender-equality paradox is alive and well, but in a form much more complex than we’d thought. Ads from male-dominated and mixed-gender sectors were comparable in both warmth and competence frames, meaning employers did not target males any differently than they normally would when targeting all genders. Ads from female-dominated sectors, on the other hand, had more warmth frames and, more alarmingly, fewer competence frames. To put it bluntly, males in male-dominated sectors are not expected to be any more competent than average but females in female-dominated sectors are expected to be warmer while simultaneously being perceived as less competent.
These findings come across as discouraging. Women in egalitarian societies are placed in a box of their own making and the numbers show it. We can argue about internalization or gender differences, but let’s reframe the issue: if there’s ever a time we should examine our biases, it’s right now – before the machines learn them.
Comments